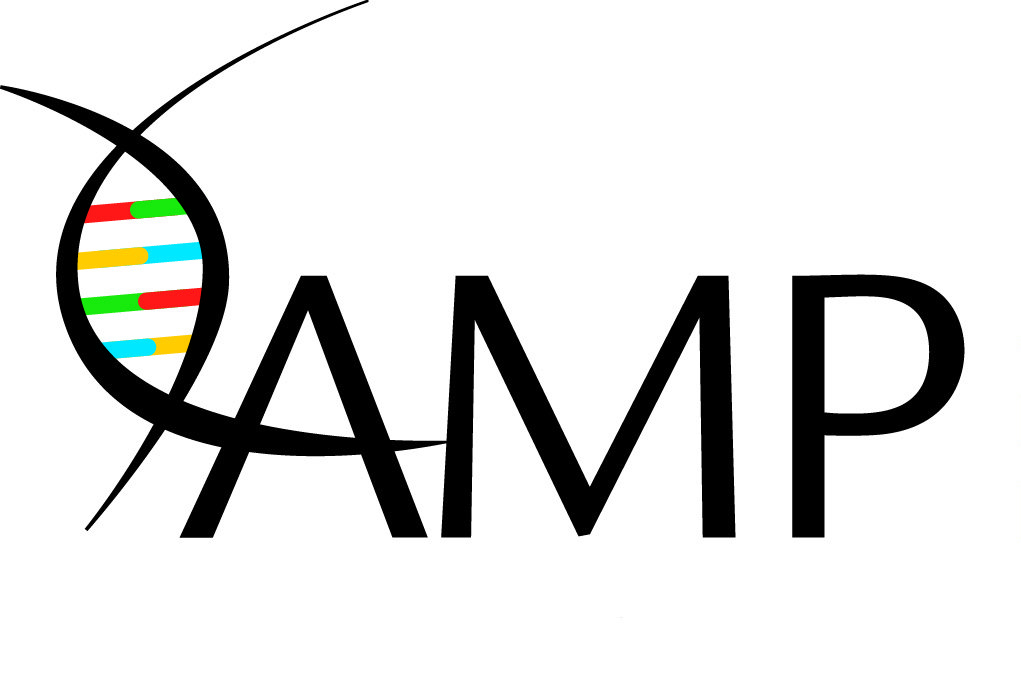
Application of an advanced data analytics platform to identify RNA biomarkers from publicly available data
November 2024 Poster Presentation
Cheryl L. Sesler1, Lukasz S. Wylezinski1,2, Mahesh B. Rao1, Guzel I. Shaginurova1, Austin M. Hilvert1, Elena V. Grigorenko1, Franklin R. Cockerill, III1,3,4, Charles F. Spurlock, III1,2,5
1 Decode Health, Nashville, TN
2 Department of Medicine, Vanderbilt University School of Medicine, Nashville, TN
3 Department of Medicine, Rush University Medical Center, Chicago, IL
4 Trusted Health Advisors, Scottsdale, AZ
5 Wagner School of Public Service, New York University, New York, NY
Introduction. Exploration of RNA sequencing (RNA-seq) data with advanced analytics leveraging artificial intelligence (AI) and machine learning (ML) is transforming the understanding of complex disease. AI-powered methods that interrogate large disparate datasets can improve biomarker discovery for diagnostic and therapeutic applications. One challenge in developing accurate and generalizable AI/ML models is the need for large, diverse sample datasets. Here, we present how a scalable advanced analytics platform can be leveraged to analyze publicly available RNA-seq data to identify disease-specific putative biomarkers.
Methods. RNA-seq data from raw to fully quantified files were obtained from NCBI databases. Raw sequences were aligned to the human genome and quantified. Differential gene expression analysis identified blood-based candidate biomarkers differentiating critical (n=12) and non-critical (n=12) COVID-19 patients. Advanced methods, including competitive ML, were performed in an expanded study identifying biomarkers that differentiate patients admitted to the emergency department (ED) with clinically adjudicated sepsis and confirmed bloodstream (n=56) or peripheral site infection (n=65) compared to non-septic ED patients (n=80). The biological relevance of candidate biomarkers was assessed through pathway enrichment analysis.
Results. We describe two distinct projects highlighting the adaptability of an advanced data analytics platform using publicly available data. In a proof-of-concept study, we identified ~650 significant genes with greater than four-fold differential gene expression between critical and non-critical COVID-19 patients. These genes were associated with biological pathways previously investigated in COVID-19 severity, including epithelial cornification, leukotriene biosynthesis, and positive regulation of small ubiquitin-related modifier transferase activity. In a larger study, quantification data were used to generate ML models to distinguish septic patients with bloodstream infection from non-septic patients with ≥ 80% accuracy using as few as five genes. The top sepsis biomarkers were associated with leukocyte activation and response to external biotic stimulus, both previously described sepsis pathways.
Conclusions. An advanced data analytics platform has been developed to accelerate biomarker discovery. This framework accommodates diverse publicly available RNA-seq datasets and can be implemented in clinical diagnostics and therapeutic target discovery. This adaptable and flexible platform permits the integration of disparate biological, clinical, and environmental datasets agnostic to disease, cohort size, and enrichment dataset type, allowing opportunities to accelerate and streamline biomarker discovery research.